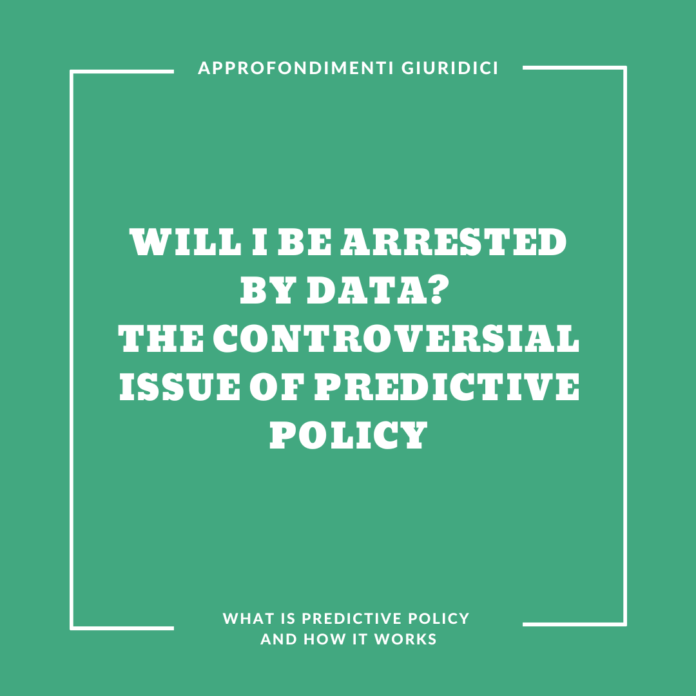
Decisions are increasingly often made by machines. In the modern world it has become very easy to see how the decisive action of man begins to be replaced by that of technology in different aspects of society, such as in financial, medical and even legal fields.
This substitution is almost always perceived negatively by the common opinion, which sees human work replaced by a machine, and tends to justify this choice as unnecessary, risky.
However, what would happen if this decision were taken to improve our security? What if a simple data analysis could prevent even just one crime within a specific urban area and make us feel safer? Will we still tend to be so critical about this the so-called “machine”?
Predictive justice plays a particularly important role in this regard, and despite conflicting opinions it is found to be an increasingly considered and debated emerging topic among security experts.
In some large cities in the United States, police departments have experimented a solution to be able to anticipate crime through computer systems that allow a large amount of data to be analyzed and based on the results, decide where to put roadblocks to identify risky subjects.
Identification is one of the key parts of the process: focusing on a particular stereotype or recurring event, which could constitute a hazard, and which should therefore be eliminated as soon as possible. At this point a potential disadvantage of predictive justice is clear. As noted by Solon Barocas in one of his research studies if the personnel involved in the investigation are not sufficiently attentive “Data mining can reproduce existing patterns of discrimination, inherit the bias of previous decision makers, or simply reflect widespread prejudices that persist in society.“[1]. In fact, there were 17 civil rights organizations that jointly expressed concern about this new use of technology, which could lead to racist outcomes, lack of transparency, accountability, accuracy, and violation of privacy.[2]
Therefore, the express consideration of race would not even be necessary to create an impact of a discriminatory and racist nature,[3] since a data-mining system already contains within it a series of decisions taken by man that can create discriminatory outcomes, also sine volunctas.[4]
In the first part of the paper, after examining the three types of predictive justice: area-based, event-based and person-based predictive policies, we will proceed to the analysis of the practice and of the respective damages and benefits that may derive from it.
In the second part will be given examples of the Italian, Dutch, French and American experience, in order to note how the European scenario can differ not only from the American one, but also in the same community, between the respective member states. Finally, space will be given to the debate on civil rights, the issues that remain open and highly criticized in order to arrive at a possible solution despite numerous oppositions.
What is predictive policy?
While the use of statistics in law enforcement is no longer a major novelty for the contemporary system, the vanguard of the world of Big Data is not only changing this position, but we could say it is also changing the face and behavior of law enforcement. As technology is progressing, a new police strategy came into effect, the so-called: predictive policy. Predictive policy is the use of predictive analytics based on mathematical models and other analytical techniques within the law enforcement system to identify potential criminal activity. Predictive policing uses computer systems to analyze large data sets that will then lead it to decide where to actually deploy the police, or to identify individuals who are presumably more likely to commit or be victims of a crime.[5] Predictive policing is the combination of predictive algorithms, information technology and criminological theories,[6] It is “the use of data and analytics to predict crime.”[7]
This practice, although it found concrete application only in the late 2000s, was first defined in the 1920s by Ernest Burgess of the Chicago School of Sociology who calculated the estimated probability of recidivism. With the shift towards predictive justice, departments found themselves having to push crime rates even lower. Since simple crimes had already been intercepted and/or prevented, it was therefore necessary to refine the application of the law through a new means.[8]
The concept of PP differs according to its objectives and, while needing targeted units for crime prevention, can be divided into three subdivisions: a) Area-based Policing b) Suspect-based Policing and c) Person-based Policing.
Area-based policing
Area-based predictive Policing is the most common type of predictive justice, but although it is the first version in analysis it is important to recognize that this type is not structurally different from the others. Taking into account the negative aspects of one is necessary to understand where and how all three types of predictive justice can go wrong despite the impacting damage ultimately differs in form and degree.
Site-based predictions are focused on the detection of so-called hotspots, places that could be the scene of future crimes, individualized by using environmental criminology acquisitions.[9] Here, it would be necessary to put more agents in direct proportion to the probability of crime verification. Algorithms, therefore, would look at the link between places, events and historical crime data, and based on this would predict the exact places where crimes would occur more easily. In some rare cases, it might even be possible to prevent the next instance of the criminal offence, but in most of them we do not have such specific tools.
The use of AI-based software has made it possible to capture and process a large amount of data, which has led to a quantum leap in these techniques. Two examples of predictive police software based on hotspots are Risk Terrain Modeling (RTM), a specific system for the prosecution of drug offences, and Predpol, a software developed by UCLA and widely used for some years in the United States and the United Kingdom, aimed at identifying hotspots in relation to a higher number of crimes.
Suspect-based policing
This system based on suspicion, can be seen as the digital derivation of criminal profiles. It is used to create an ex-nihilo model of what a person might commit and what plot a crime might have.[10] Based on this model, the suspects will be identified through a research and comparison investigation.
This is therefore the most worrying of the three types since there is a risk that racial disparities in the result of the algorithm will create a greater degree of suspicion and greater likelihood of finding a probable cause because of the race of a suspect, but we’re going to examine this risk later in the paper.
Person-based predictive policing
The last type of predictive justice is based on the person, but not on investigation. This is done by combining their personal information such as age, gender, marital status, substance abuse stories, criminal precedents etc. Intrado’s Beware software, for instance, allows police to tap into publicly available data, including social media data, to check the “threat score” of a person or address when a 911 call arrives, and a green, yellow or red label is assigned depending on the severity.[11] Since the effects of these systems are aimed at individuals,[12] the damage also seems different from the results of resource management decisions driven by crime mapping but even though some of the effects can be similar in scale. As reported by the sociologist Sarah Brayne from the University of Texas, the Los Angeles Police Department’s predictive police uses a simple system based on scores, where the number of points is directly proportional to the threat posed by the person.[13]
Among them, the person-based PP is by far the most controversial, since it specifically identifies names and faces individually, and can be applied to different types of crime such as mass shooting attacks[14], terrorism and financial crimes, but also on matters regarding the community policing.
Predictive policy in practice
As we have seen above, the most common form of predictive justice is location-based policing, which takes the retrospective data of crime, and applies them in perspective to determine its distribution. An example is a neighborhood or a specific area of the city where robberies occur frequently at night. On the basis of a probability calculation based on repetition, the more an event occurs in a given area, the more likely it is to occur again, so, it is logical to intervene with the police. This intervention could consist in the simple placement of an agent at the complex of houses in order to prevent future invasion. Nevertheless, it is necessary to take into account the fact that this decision may not lead to a permanent solution, but only to a shift of the hotspot of crimes that can be committed. The other form of person-based predictive policing law enforcement may predict individuals or groups that are more likely to be involved in crimes, either as victims or offenders.
In both forms of PP there are four key steps.[15]
The first of these is the collection of data that can vary from basic data on crime (for example, when and where historical crimes occurred), to more complex environmental data such as seasonality, neighborhood composition or risk factors (for example, empty lots, parks, ATMs). The second phase involves the analysis of previously collected data and provides forecasts on future crime. However, it is necessary to clarify that before deciding which predictive method is to be use, law enforcement is required to consider not only the type of crime they want to target but also the resources of their department.
The third phase of the predictive cycle is the direct police action. This part implicitly implies a precedent one, which is the distribution of the predictions of the crime to the commanders, and then their concrete intervention with the deployment of officers on the ground. When patrol officers do not have to answer to service calls, they tend to take care of the surveillance of people and places that according to the models analyzed could be susceptible to future crimes.
Finally, the fourth phase, target response, highlights that these predictive police sequence, or ‘battle rhythm’ as it is called by some law enforcement officials, grows increasingly complex over time. Law enforcement agencies must account for individual responses to police intervention. As stated above, the intervention could either deter and thus prevent Actus reus or could lead to the relocation of crime to another area.
Benefits
Although this is a new and still widely debated practice, it is necessary to recognize that there are positive aspects that have relied on cities applying the algorithm in crime prevention. Among the points in favor of predictive policy is certainly the effective reduction of crime, and a large number of studies support this cause. In the city of Santa Cruz, California, the use of the algorithm led, in a period of six months, to the reduction of burglaries to – 19%, in a location of 500-square-foot.[16] The same thing was reproduced in the city of Los Angeles where hotspot maps were distributed to the officers without informing if they were created with official methods such as LAPD, or by the algorithm. The result was that the algorithm provided double the extra precision, and while crime in general had increased by 4% across LA, in Foothills, where the algorithm had been applied, it had decreased by 12%.
The second positive aspect of predictive Policing is that, according to its supporters, it would lead to more objective decisions, diverting public officials from making arbitrary decisions based on bias rather than evidence. To support this was the US General Attorney who was able to remove Camden, New Jersey, from the record of the most dangerous city in America, reducing murders by 41% and the general rate by 26%.
By promoting the decision-making process from objective data and evidence, some discrepancies in law enforcement would be alleviated. Returning to the previous example, when the crime map was compiled at LA, it was not based on human prejudice, whereas in the traditional method this inevitably happened. Therefore, algorithms may really be able to offer more accurate prevention, determining the identity of the attackers and identifying the vulnerability of the assaulted.
This position is however a double-edged sword since as we will see later, the alleged objectivity of the PP is also one of the main criticisms since total efficiency is achieved only if the algorithm is stripped of any form of bias and prejudice.
Drawbacks
As mentioned above, the two main types of predictive policy applied are area-based and individual-based. However, while technology-focused businesses[17] are only focusing on the positive aspects of AI-based PP, rights-focused NGOs are arguing for possible civil rights violations. [18]
Recent studies have identified numerous negative impacts of PP, which in some cases even pushed cities that had initially accepted this practice, to ban it while respecting the protection of their citizens.[19]
The first problem that arises is that some data may be too personal to be stored, and those who have it, whether for lack of ability or professionalism, may fail to fulfill this task. Information collected and stored by a police department often risks leaking due to an economic factor, since data security is costly in terms of training and personnel. Considering the sensitive nature of this information, the possibility of data loss is particularly alarming, and thus the failure to respect citizens’ right to privacy. It should also be remembered that the prompt availability of a lot of personal data on social media aggravates this situation, and that the consequences will be increasingly significant as more and more personal data are disclosed and collected in public without precise expectations of privacy.
In addition to the problems of privacy, considering the principle of presumption of innocence, that every individual is considered innocent until proven guilty, while predicting the propensity to crime, this principle is necessarily undermined.
It may be noticed how simple it is to fall into subjectivity, and how predictive vigilance ends up depending also on the integrity of its implementors. Decades of criminological research claim that the documents reported by the police are often the result of what they see rather than an objective recording of what is happening, and where the spotlight is aimed at minority groups, it easily creates a vicious circle filtered by the lens of racism. Crime statistics collected on the basis of a racist policy will create racist predictions, leading to an excess of policing that will continue to generate misleading data and racist predictions.
Another drawback besides the lack of accuracy is therefore that it can produce biased results which, seeing police suspicion concentrated on some areas over others, and a more natural propensity to search for criminals in these hotspots, they would create a community distrust of their own law enforcement. This systemic collateral damage has far greater impacts than you can imagine.
To summarize, it is therefore necessary to recognize how the promise to improve the social system and to remedy crime immediately, leads to serious violations and defects. The factors regarding privacy, lack of accuracy, discrimination and accountability are just a few of them, and for the moment, the attempt to create a fair and transparent algorithmic decision-making process is still imperfect.
The Italian experience: the KeyCrime predictive policy algorithm and eSecurity predictive urban security in Trento
In Italy, in the last 15 years, the police detective Mario Venturi has developed KeyCrime[20], a predictive police software based on an algorithm for the analysis of criminal behavior. The system would be able to screen about 11000 variables for each type of crime committed: from time, place and appearance, to the accurate reconstruction of witnesses, suspects, video material and the criminal’s modus operandi.[21] This would greatly facilitate the fight against future crimes.
At the base of the project of the Italian policeman there is the idea of making the use of the force of the police officers, more rational, and avoiding a mass of agents in areas that do not require it. This is not done by adopting a place-based predictive policy, but by focusing on the suspected individual so that it is easier to catch him.[22]
An additional and unique feature of KeyCrime is that it is able to attribute a number of crimes to the same suspect, and if the number of crimes is greater than four, the efficiency of the algorithm is 9% higher than the traditional method. This software has been particularly successful in the Lombard city of Milan, where according to data there has been a 23% crime reduction in pharmacy robberies, and a 70% efficiency in identifying suspects in bank robberies.[23][24]
In the Italian scenario another initiative deserves to be mentioned, namely the creation of the first experimental laboratory of predictive urban security defined as eSecurity[25], co-funded by the European Commission and coordinated by the research group of the Faculty of Law of the University of Trento with the ICT Centre of the Bruno Kessler Foundation, the Department of Trento and the Municipality of Trento.
The project, based on the theory of hot spots and models adopted by common law countries, employs algorithms that can: use past georeferenced crimes and smart city data; consider the concentration of victimization, urban insecurity and disorder at the city level; and predict the place, time and reason why certain types of deviance will occur. They are therefore explicitly aimed at providing data-based information to policy makers for smart and effective urban security planning.
The US experience: the controversial Loomis case
For a long time now, the United States has been using predictive algorithms both in the pre-trial phase for the determination of the bail, and in the pre-decision phase for the evaluation of the possible definition of the procedure with a “probation judgment” or testing of the subject.
The case that sparked more discussion in 2016 was the Loomis case, presented by the Supreme Court of Wisconsin in which the mechanisms of AI were also applied in the cognition phase.
In 2013, Loomis was driving a car previously used for a shooting in the state of Wisconsin, USA. Stopped by the police, he was charged with five charges, all of which were repeated: 1) endangering security, 2) attempting to escape or evade a traffic officer, 3) driving a vehicle without the owner’s consent, 4) possession of a firearm by a convicted felon, 5) possession of a short-barreled rifle or pistol. The defendant agreed to settle the penalty for the less severe charges sub-2) and 3). After accepting Loomis’ admission of guilt, the Court ordered a Presentence Investigation Report (PSI), that is, a report of the results of the investigations conducted on the personal history of the accused, preliminary to the sentence on the determination of the penalty, the aim is to verify the presence of circumstances useful to modulate the severity of the same. This investigation is based on a series of 137 questions related to the subject, the results of which are elaborated by the COMPAS software, in order to determine both the danger and the possibility of repetition of the crime. The defendant was therefore arrested and sentenced to six years, with the justification that the penalty was not based only on the fact contested, but also on the score given to the defendant by COMPAS.
COMPAS is an assessment tool that serves to predict the risk of relapse, and together to identify the needs of the individual in areas such as employment, availability of housing and drug abuse. The algorithm shall process the data obtained from the defendant’s file and his replies obtained following an interview. This is followed by a graph with three bars representing on a scale of 1 to 10 the risk of pre-trial relapse, the risk of general relapse and the risk of violent relapse. The risk scores are intended to predict the general probability that individuals with a similar criminal history are more or less likely to commit a new crime once they are released. COMPAS therefore, would not foresee the risk of individual recurrence of the accused but would elaborate the forecast comparing the information obtained from the individual with those relating to a group of individuals with similar characteristics.
Loomis allegedly contested the fact that the defense was not given the opportunity to examine the criteria by which the software had determined the dangerousness of the subject because the software is secret and protected by copyright. In short, the defense would not be guaranteed a fair trial.
The appeal reached the Wisconsin Supreme Court, which upheld the conviction, arguing that the verdict would be the same even without the use of Compas.
It was precisely as a result of this case that the American non-profit organization ProPublica spread a very detailed analysis on the software “Compas”.
This analysis revealed not only a lack of transparency as to its operation, but also that the data produced by the program led to discriminatory results of the target population. The software, for example, elaborated a higher probability of relapse for black subjects than whites. In particular, blacks would have had 77% more chances to repeat violent crimes such as murder, rape, robbery and aggravated assault.
The media power that affected this case drew attention to the ethical, moral and legal implications of the use of artificial intelligence mechanisms applied to the process, the appreciable repercussions on the rights and freedoms of persons deriving from the mathematical model underlying the design of the algorithm, the definition of inputs and the processing of correlative outputs.
Following this case, the topic of algorithm in predictive justice was reopened by the Pennsylvania Government Commission to design an algorithm that would assess the risk of recurrence after the subject was convicted. The idea of the project was to compare known stakeholder information with responses made with statistics describing the characteristics of known offenders, possibly provided by the county’s probation departments.
As you can imagine there were many protests, especially in defense of civil liberties. The American Civil Liberties Union, which was the spokesman for these criticisms, denounced mainly: the lack of transparency of the logic used and the inevitable increase in racial prejudice or referring to the minority belonging to the person concerned.
Although algorithms continue to be used in much of the United States, several organizations have emerged such as the Media Mobilizing Project of Philadelphia creator of a database that analyzes the use of prediction algorithms throughout the nation, through which it is analyzed how risk assessment tools are used, and how they affect the freedoms of individuals; and the Californian Silicon Valley de-bug which is dedicated to interviewing the family of each defendant, By bringing to each hearing the personal information collected in this context, sharing it with the defenders as a kind of counterweight to the “cold” application of algorithms.[26]
Europe: the central role of ethics and human dignity
The US experience opens the way for intense reflection on the role of artificial intelligence applied to the process, with particular reference to the implications on the rights and freedoms of individuals.
With the Convention on the Protection of Individuals with regard to Automated Processing of Personal Data, In Strasbourg on 28 January 1981 the Member States of the Council of Europe sought to ensure that the fundamental rights and freedoms of every person in respect of automated processing were respected. This principle was subsequently reaffirmed by the Protocol of October 2018, where in the preamble the Member States of the Council of Europe stress the need “to ensure the human dignity and protection of the human rights and fundamental freedoms of each individual and, in view of the diversification, intensification and globalization of data processing and the flow of personal data, personal autonomy based on the right of a person to control his personal data and the processing of such data”. In Article 1 they clearly state that the purpose of the Convention is to:
“to protect every individual, whatever their nationality or residence, in relation to the processing of their personal data, thereby contributing to the respect of their human rights and fundamental freedoms, in particular the right to privacy”[27].
The primacy of human rights and dignity has been reaffirmed by the Committee of Ministers of the Council of Europe, and the general principles on which artificial intelligence in the field of justice should be based are as follows:
- the additional role of “digital” access to justice over analogue access to facilitate better access to court rulings and chancelleries for both citizens and legal professionals
- the prohibition of discriminatory effects of the algorithms applied in the field of justice that may violate the principles dictated in the field of privacy and personal data protection
- the right of each citizen to have recourse in any case to the judicial control of a judge in order to obtain an individual decision
- the use of digital tools should respect the principles of due process, the secrecy of investigations and the principle of knowledge and transparency of judicial decisions
- the use of such tools should prevent the dissemination of illegal content and false news, which could create a serious impact on democratic societies, while ensuring freedom of expression and information.
Conclusion
The last twenty years have seen a wide use of data-driven policies, practices and technologies in the public sector in order to reduce dependence on subjective factors and to react more objectively to social, economic and political issues. However, increasing dependence on data presents serious risks for equity, fairness and justice, if the practices behind data creation, review and maintenance are not closely monitored.
The study of some cases, as seen in the previous paragraphs, highlights the risks and consequences associated with over-reliance on irresponsible and potentially distorted data to address sensitive issues such as public safety.
Therefore, it would seem that police departments, in the absence of explicit requirements and incentives, would self-control and reform those activities that create distorted data, and therefore external supervision by an independent authority would be necessary.
Assuming that predictive analysis through the algorithm can be very useful for the suppression of crimes, however there is a need to find a balance between the need for effective law enforcement and crime prevention, and the rights of individuals.
In my opinion, it would be necessary to draw up a specific regulation for this particular investigative technique, as well as an independent authority that controls, firstly, the acquisition of data, authorizes its use and, in the long term, verifies police operations. That authority should also be able to penalize officials in the event of discriminatory practices detrimental to the constitutional damage of the person.
It is appropriate to develop technical measures to ensure algorithmic accountability and transparency in order to avoid negative consequences for the right to privacy and discrimination issues. Only compliance with these guarantees can eliminate, or at least reduce, compliance of predictive policing practices with the protection of civil rights.
In conclusion, I personally believe that predictive analytics is not a blunt tool for targeting criminals but instead could be used to identify social needs and economic problems affecting those areas with a high crime rate.
For this reason, despite the many criticisms, I believe that there can also be benefits at different levels: firstly, it would facilitate law enforcement to define critical areas, allocate resources as effectively as possible at any given time, to intervene at operational level with initiatives aimed at preventing and eliminating criminal phenomena and constantly measuring the results achieved; secondly, it would help local authorities to discover the extent of the phenomena and their nature, in order to develop more effective policies and measures in the field of crime and public security and to monitor their results.
As a result, the point is not only to put rules that break this data of discrimination, but rules that are not only on the production of technology, but also on the use of it, since we never forget that before the algorithm the human factor is not the weakest, it is the only one that counts.
Bibliography
- (2020, April 1).Predictive policing explained. Brennan Center for Justice. Retrieved November 12, 2022, from https://www.brennancenter.org/our-work/research-reports/predictive-policing-explained
Algorithms and Autonomy Ethics Automated Decision Systems | Law and … (n.d.). Retrieved November 12, 2022, from https://doi.org/10.1002/asi.24651
Carrer, S. (2019, April 29). Se l’amicus curiae è un algoritmo: Il chiacchierato Caso Loomis Alla Corte Suprema del Wisconsin. Giurisprudenza penale. Retrieved November 12, 2022, from https://www.giurisprudenzapenale.com/2019/04/24/lamicus-curiae-un-algoritmo-chiacchierato-caso-loomis-alla-corte-suprema-del-wisconsin/
Colpo al supermarket previsto dal computer: Due arresti. Corriere della Sera. (2015, March 8). Retrieved November 12, 2022, from https://milano.corriere.it/notizie/cronaca/15_marzo_08/colpo-supermarket-previsto-computer-due-arresti-c907f418-c571-11e4-a88d-7584e1199318.shtml
Council of Europe: Convention for the Protection of individuals with regard to automatic processing of personal data. (1981). International Legal Materials, 20(2), 317–325. https://doi.org/10.1017/s0020782900032873
Giustizia Predittiva: Il Progetto (concreto) della corte D … – altalex. (n.d.). Retrieved November 12, 2022, from https://www.altalex.com/documents/news/2019/04/08/giustizia-predittiva
Giustizia predittiva: La dignità umana faro per l’ai Nei processi. Agenda Digitale. (2020, November 17). Retrieved November 12, 2022, from https://www.agendadigitale.eu/cultura-digitale/giustizia-predittiva-la-dignita-umana-faro-per-lai-nei-processi/#post-79790-footnote-ref-2
Hardt, M. (n.d.). Approaching Fairness in Machine Learning. Approaching fairness in machine learning. Retrieved November 12, 2022, from http://blog.mrtz.org/2016/09/06/approaching-fairness.html
Hung, T.-W., & Yen, C.-P. (2020). On the person-based predictive policing of ai. Ethics and Information Technology, 23(3), 165–176. https://doi.org/10.1007/s10676-020-09539-x
Italy. AlgorithmWatch. (n.d.). Retrieved November 12, 2022, from https://algorithmwatch.org/en/automating-society-2019/italy/
La Chiave del crimine – poliziadistato.it. (n.d.). Retrieved November 12, 2022, from https://www.poliziadistato.it/statics/16/la-chiave-del-crimine.pdf
La Giustizia predittiva approda su one legale con la nuova … – altalex. https://www.altalex.com/documents/news/2021/09/14/giustizia-predittiva-stato-arte-italiano-internazionale. (n.d.). Retrieved November 12, 2022, from https://www.altalex.com/documents/news/2021/09/08/la-giustizia-predittiva-approda-su-one-legale-con-la-nuova-funzionalita-giurimetria
Mendroca, P. (2022, October). Cybersecurity and globalisation . University conference at NOVA. Lisbon; Nova School of Law.
Morelli, F. (n.d.). Alla Scuola sant’anna di pisa si allena l’algoritmo che prevede Le Sentenze. Ristretti. Retrieved November 12, 2022, from https://ristretti.org/index.php?option=com_content&view=article&id=100733%3Aalla-scuola-santanna-di-pisa-si-allena-lalgoritmo-che-prevede-le-sentenze&catid=220%3Ale-notizie-di-ristretti&Itemid=1
A national discussion on predictive policing – Office of Justice Programs. (n.d.). Retrieved November 12, 2022, from https://www.ojp.gov/pdffiles1/nij/grants/230404.pdf
Platform, E. L. (n.d.). 4 benefits and 4 drawbacks of Predictive Policing. Liberties.eu. Retrieved November 12, 2022, from https://www.liberties.eu/en/stories/predictive-policing/43679
Predictive Policing – Data & Civil Rights Conference. (n.d.). Retrieved November 12, 2022, from http://www.datacivilrights.org/pubs/2015-1027/Predictive_Policing.pdf
Predictive policing today: A shared statement of civil rights concerns. (n.d.). Retrieved November 12, 2022, from http://civilrightsdocs.info/pdf/FINAL_JointStatementPredictivePolicing.pdf
Rubel, A., Pham, A., & Castro, C. (1970, January 1). Alan Rubel, Adam Pham & Clinton Castro, agency laundering and algorithmic decision systems. PhilPapers. Retrieved November 12, 2022, from https://philpapers.org/rec/RUBALA
Sabelli, C. (2017, December 11). Scacco alla malavita, arriva l’algoritmo Che Prevede I reati. Il Messaggero. Retrieved November 12, 2022, from https://www.ilmessaggero.it/primopiano/cronaca/algoritmo_reati-3420795.html
Santucci, G. (2018, April 10). Milano, il programma anti rapine diventa una startup della sicurezza. Corriere della Sera. Retrieved November 12, 2022, from https://milano.corriere.it/notizie/cronaca/18_aprile_10/milano-programma-anti-rapine-diventa-startup-sicurezza-a355ba22-3c81-11e8-87b2-a646d975b0f5.shtml
Selbst, A. D. (2016, October 1). Disparate impact in Big Data Policing. SSRN. Retrieved November 12, 2022, from https://papers.ssrn.com/sol3/papers.cfm?abstract_id=2819182
Selbst, A. D. (2016, October 1). Disparate impact in Big Data Policing. SSRN. Retrieved November 12, 2022, from https://papers.ssrn.com/sol3/papers.cfm?abstract_id=2819182
SocialismoItaliano1892E’ un progetto che nasce con l’intento “ambizioso” di far conoscere la storia del socialismo italiano (non solo) dei suoi protagonisti noti e meno noti alle nuove generazioni. Facciamo comunicazione politica e storica. (2021, July 30). Il Caso Loomis –. Socialismo Italiano 1892 – Il Portale Informativo di Socialismo XXI. Retrieved November 12, 2022, from https://www.socialismoitaliano1892.it/2021/07/30/il-caso-loomis/
[1] See Solon Barocas & Andrew D. Selbst, Big Data’s Disparate Impact, 104 CALIF. L. REV. 671, 674 (2016). Throughout this Article I will focus on racial discrimination, primarily because that is the focus of the broader discrimination discussion as it pertains to policing. The arguments about data and discrimination apply equally well to other classes of vulnerable populations based on gender, gender identity, sexual orientation, as well as non-legally protected classes such as social class. See generally id.
[2] THE LEADERSHIP CONFERENCE ON CIVIL & HUMAN RIGHTS ET AL., PREDICTIVE POLICING TODAY: A SHARED STATEMENT OF CIVIL RIGHTS CONCERNS (Aug. 31, 2016) [hereinafter STATEMENT OF CIVIL RIGHTS GROUPS]
[3] Historically, the naive approach to fairness has been to assert that the algorithm simply doesn’t look at protected attributes such as race, color, religion, gender, disability, or family status. So, how could it discriminate? This idea of fairness through blindness, however, fails due to the existence of redundant encodings. There are almost always ways of predicting unknown protected attributes from other seemingly innocuous features.”. Moritz Hardt, Approaching Fairness in Machine Learning, MOODY RD (Sept. 6, 2016)“
[4] See Barocas & Selbst, supra note 19, at 673–74.
[5] 4 Benefits And 4 Drawbacks Of Predictive Policing. (s.d.). Liberties.eu.
[6] Georgia Law Review. (n.d.). Retrieved November 12, 2022, from https://georgialawreview.org/
[7] Bachner, supra note 8, at 6; see also CRAIG D. UCHIDA, NAT’L INST. OF JUSTICE, NO. NCJ 230404, A NATIONAL DISCUSSION ON PREDICTIVE POLICING: DEFINING OUR TERMS AND MAPPING SUCCESSFUL IMPLEMENTATION STRATEGIES 1 (2009), (“Predictive policing refers to any policing strategy or tactic that develops and uses information and advanced analysis to inform forward-thinking crime prevention.” (emphasis omitted)).
[8] Predictive Policing – Data & Civil Rights Conference. (n.d.). Retrieved November 12, 2022
[9] F. BASILE, Intelligenza artificiale e diritto penale: quattro possibili percorsi d’indagine in Diritto penale e uomo, 10, 2019, p.11
[10] Michael L. Rich, Machine Learning, Automated Suspicion Algorithms, and the Fourth Amendment, 164 U. PA. L. REV. 871, 876 (2016) (describing “Automated Suspicion Algorithms” that “seek to predict individual criminality”).
[11] Justin Jouvenal, The New Way Police Are Surveilling You: Calculating Your Threat
‘Score,’ WASH. POST (Jan. 10, 2016), https://www.washingtonpost.com/local/public-safety/the-n ew-way-police-are-surveilling-you-calculating-your-threat-score/2016/01/10/e42bccac-8e15-11e 5-baf4-bdf37355da0c_story.html.
[12] John Buntin, Social Media Transforms the Way Chicago Fights Gang Violence, GOVERNING (Oct. 2013), http://www.governing.com/topics/urban/gov-social-media-transforms-c hicago-policing.html (describing the use of social media data in policing techniques in Chicago); Johnson et al., supra note 8 (explaining that the use of social network analysis is “a valuable tool for law enforcement”); Craig Timberg & Elizabeth Dwoskin, Facebook, Twitter And Instagram Sent Feeds That Helped Police Track Minorities In Ferguson And Baltimore, Report Says, WASH. POST (Oct. 11, 2016), https://www.washingtonpost.com/news/the-switch/ wp/2016/10/11/facebook-twitter-and-instagram-sent-feeds-that-helped-police-track-minorities- in-ferguson-and-baltimore-aclu-says/.
[13] Sarah Brayne, Big Data Surveillance: The Case of Policing, 82 AM. SOC. REV. 977, 986–89 (2017).
[14] According to USA TODAY (Baig 2019), after the Parkland shoot- ing, three US companies (Bark Technologies, Gaggle.Net, and Securly Inc.) claim that their AI systems can detect possible signs of cyber bullying and violence by scanning student emails, texts, docu- ments, and social media activity.
[15] Based on Price, M. et al., 2013. National Security and Local Police. New York: Brennan Center for Justice.
[16] Sanfilippo, M. R. (2022). Algorithms and autonomy: The ethics of automated decision systems. RubelAlanCastroClintonPhamAdamCambridge University Press, 2021. 206 pp. £ 29.99 (paperback). (9781108795395). Journal of the Association for Information Science and Technology. https://doi.org/10.1002/asi.24651
[17] For example, Israel’s Faception and the UK’s Wesee
[18] For example, Amnesty International UK 2018; Human Rights Watch 2017, 2019
[19] The Los Angeles police department has been a pioneer in predictive policing, for years touting avant-garde programs that use historical data and software to predict future crime. But newly revealed public documents detail how PredPol and Operation Laser, the department’s flagship data-driven programs, validated existing patterns of policing and reinforced decisions to patrol certain people and neighborhoods over others, leading to the over-policing of Black and brown communities in the metropole. https://www.theguardian.com/us-news/2021/nov/07/lapd-predictive-policing-surveillance-reform
[20] La Chiave del crimine – poliziadistato.it. (n.d.). Retrieved November 12, 2022, from https://www.poliziadistato.it/statics/16/la-chiave-del-crimine.pdf
[21] Santucci, G. (2018, April 10). Milano, il programma anti rapine diventa una startup della sicurezza. Corriere della Sera. Retrieved November 12, 2022, from https://milano.corriere.it/notizie/cronaca/18_aprile_10/milano-programma-anti-rapine-diventa-startup-sicurezza-a355ba22-3c81-11e8-87b2-a646d975b0f5.shtml
[22] Colpo al supermarket previsto dal computer: Due arresti. Corriere della Sera. (2015, March 8). Retrieved November 12, 2022, from https://milano.corriere.it/notizie/cronaca/15_marzo_08/colpo-supermarket-previsto-computer-due-arresti-c907f418-c571-11e4-a88d-7584e1199318.shtml
[23] Ibidem
[24] Sabelli, C. (2017, December 11). Scacco alla malavita, arriva l’algoritmo Che Prevede I reati. Il Messaggero. Retrieved November 12, 2022, from https://www.ilmessaggero.it/primopiano/cronaca/algoritmo_reati-3420795.html
[25] https://www.esecurity.trento.it
[26] J. Kourkounis, New York Times
[27] (1981), C. f. t. P. o. I. w. R. t. A. P. o. P. D. (1981)p.7). Convention for the Protection of Individuals with Regard to Automatic Processing of Personal data =: Convention pour la protection des personnes a l’égard du traitement automatisé des données a caractère personnel. The Council.